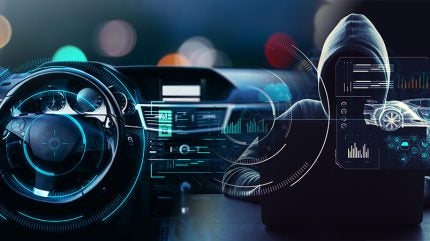
The introduction of ChatGPT is on the rise. Volkswagen, for example, recently said that drivers will be able to carry out back and forth dialogue in its vehicles equipped with ChatGPT-based services.
The inclusion of this technology within our vehicles allows the potential to unlock a range of capabilities related to navigation, managing infotainment systems, getting weather or traffic updates and answering more general questions.
However, does this onboard help come with risks? Companies such as Synopsys, an electronic design automation company, say it is vital to consider the type of training data which is used to train this technology. Alongside this, policy use to define what responses and type of information are allowed is also key for automakers to consider.
We spoke to Dennis Kengo Oka, senior principle automotive security strategist executive, Synopsys, to learn more about the benefits ChatGPT can offer, and some potential risks.
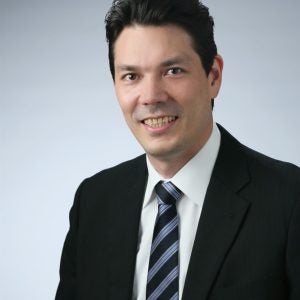
Could you provide me with some background on the company and your role?
Dennis Kengo Oka (DKO): I work globally with automotive customers such as OEMs, Tier 1s and Tier 2s, and assist them on security and associated strategic areas. More specifically, I provide guidance on how to establish secure software development platforms and secure development processes within the organisation.
How well do you really know your competitors?
Access the most comprehensive Company Profiles on the market, powered by GlobalData. Save hours of research. Gain competitive edge.

Thank you!
Your download email will arrive shortly
Not ready to buy yet? Download a free sample
We are confident about the unique quality of our Company Profiles. However, we want you to make the most beneficial decision for your business, so we offer a free sample that you can download by submitting the below form
By GlobalDataThe Software Integrity Group at Synopsys helps organisations build high-quality, secure software faster by offering application security testing tools and services. Synopsys is recognised as a leader among application security testing vendors evaluated by Gartner for the past seven years.
What are some of the benefits ChatGPT can offer?
Large-Language Models (LLMs) such as ChatGPT can support various use-cases in the automotive industry. For example, they can enable intelligent digital voice assistants to be integrated into vehicles. These voice assistants use Natural Language Processing (NLP) and speech recognition technology to understand and respond to the driver’s or passengers’ voice commands.
As such, drivers can use voice commands to control various vehicle functions, including adjusting the climate control, requesting route planning, controlling the playlist to play music, adjusting the volume and making phone calls; all achieved without physically having to press any buttons. It produces an enhanced user experience.
Moreover, these digital voice assistants can be used further to help customers easily find relevant information regarding their car without having to read through hundreds of pages of the user’s manual. For example, the driver can ask why a certain warning light on the dashboard is blinking and the digital voice assistant will provide the answer.
Besides being integrated into vehicles, LLMs can also be used to, for example, optimise the development process at automotive organisations. For example, LLMs can be used to simplify requirements management and test management. This means that LLMs can process requirements documents, verify the syntax, and sort out requirements related to hardware, software, processes and assign them to the right teams. Moreover, LLMs can generate appropriate test cases for the analysed requirements to allow for better requirements traceability. AI solutions can also help optimise the design of components in terms of performance, safety and cost, as well as improve efficiency during implementation by auto-generating code.
What are some of the potential risks?
There are numerous risks that need to be considered when using AI and LLMs. For example, a common attack vector is the prompt injection attack, where an attacker feeds the AI system with certain data to make it behave in a way that the system was not designed for. This attack can be performed in two ways: direct or indirect.
The direct approach is similar to jailbreaking in the sense that it breaks down the restrictions of the AI system prompt. This allows the attacker to directly gain access to back-end systems. In contrast, the indirect approach relies on data being entered from an outside source; for example, a website connected to the model, or a document uploaded to the AI system.
There are numerous risks that need to be considered when using AI and LLMs.
Another big concern is data privacy. Large amounts of data are collected, stored and processed by the AI system. This data may contain private or sensitive data. As such, an attacker may target this data and be able to extract it; for example, the vehicle location data or other customer data from the output generated by an AI system or LLM application.
What advice would you give to OEMs looking to integrate the technology into their vehicles?
Many OEMs are looking into creating their own AI models, which then introduces the risk of model theft. For example, if the model contains proprietary algorithms or specific IP, the model can be targeted by attackers and could potentially be copied or reverse-engineered. An attacker can then abuse the stolen model to analyse how certain functions work, or gain unauthorised access to sensitive information in the model. For example, an AI model for repair shops could contain proprietary information on how to reprogram a new key for a vehicle, or how to enter engineering mode on an ECU. An attacker targeting this model could potentially rebuild the model by reverse-engineering the features or functions that the model provides. As such, OEMs should consider what type of critical or sensitive data the AI model should be trained on and consider the risk of model theft.
Another concern when creating your own AI model is managing the data that the model is trained on. For example, an attacker can target modifying the behaviour of the AI model through a so-called ‘training data poisoning attack’. In this scenario, the attacker modifies or includes certain type of malicious or incorrect data in the training data set. As such the AI model becomes tainted by being trained on this wrong data. As a result, the AI system, although seemingly working “correctly” according to the model based on the tainted training data, may actually misbehave from the intended design or behaviour.
It is worth noting that this attack requires the attacker to have access to the training data, be able to modify the training data undetected, and then ensure that the AI model is trained using this tainted training data. With supply chain attacks on the rise, this attack vector would be something OEMs should carefully consider preventing.
Another consideration is that while AI systems and LLMs provide significant benefits for numerous use-cases, it is imperative for organisations to recognise that studies have shown that generative AI systems have inaccuracies. They may generate incorrect, unsafe, or insecure content. This concept is known as “AI hallucinations” and may occur up to as much as 20% of the time. Moreover, since generative AI systems typically give their answers confidently, the challenge for users is to understand which parts of the output can be trusted and which parts of the output may be factually incorrect and potentially harmful if used ‘as is’. Thus, while using AI has major benefits, organisations need to seriously consider things like AI hallucinations and potential inaccuracies when relying on AI technologies.
What do you see this year holding for the technology?
AI technologies will continue to be more widely deployed in the automotive industry as they provide important benefits. We will see more AI solutions integrated into the vehicle but also increased usage of AI solutions during the development and operational phases of the vehicle lifecycle. For example, during development, AI solutions will help with more advanced simulation and testing, and optimization of the development processes as mentioned above.
AI technologies will continue to be more widely deployed in the automotive industry as they provide important benefits.
During the operational phases, AI will improve the efficiency of analysing large amounts of data collected from vehicles to help with data-driven decision making. This also includes detecting anomalies and potential cyberattacks on vehicles, and thus can be applied to support the continuous cybersecurity monitoring use-case.