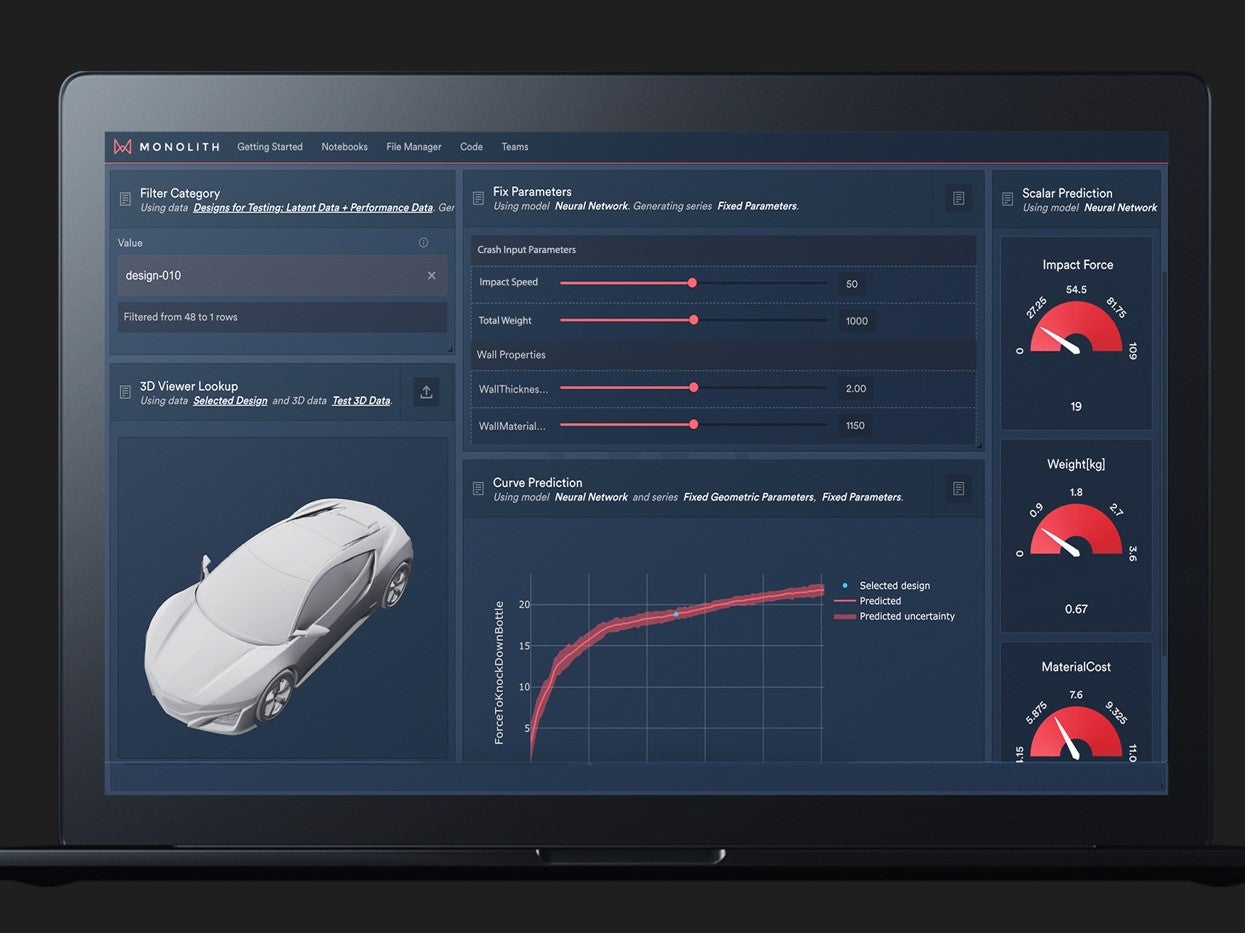
During vehicle development, lengthy tests and trials must be completed to ensure the safety, performance, and overall quality of a vehicle. Although necessary, these tests take up many hours with some needing to be restarted or altered mid-way through the process. What if there was a solution to this issue?
Software development company Monolith has partnered with BMW Group. In the partnership, the automotive giant is using AI software to accelerate the development of vehicles. Using AI technology, BMW is working towards solving highly complex challenges, as well as being able to instantly predict performance of vehicles in various situations.
We spoke to Monolith CEO and founder, Richard Ahlfeld to learn more about the technology and the capabilities that it offers those working in the automotive space.
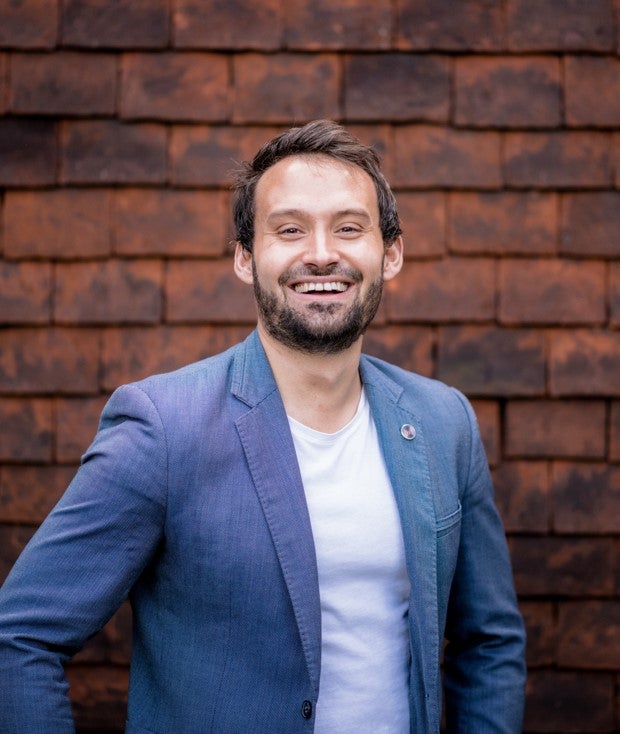
Just Auto (JA): Could you tell me a little bit about the company?
Richard Ahlfeld (RA): I’m the founder and CEO of Monolith. We have been around since 2016, but in the beginning, it was just me doing consulting work for large engineering companies. We’ve been a software company with venture capital investments since 2018.
We build software focused on the needs of engineers who work primarily in the automotive industry with sensor data. Whether that’s from an engine test, a battery or from a smart device which works with the car, engineers need to make sense of that data and better understand the performance of the product when it fails, how long it lasts, how to model it and how to calibrate it for better performance.
What does the technology offer clients in the automotive space?
I think a classic vector business model in general is software as a service. People log into a cloud software that is hosted on Amazon Web Services (AWS), they upload their test data, they engineer data sets. We have a completely coding-free user interface that allows them to build machine learning models on top of this data. That means that they can build accurate, data-driven models directly from the raw sensor data. You can understand how a system works better, and find patterns when it fails and predict how it would behave in a completely different scenario.
There are two benefits that machine learning models have compared to a more conventional approach. These data-driven self-learning models, instead of a physical model, can be more accurate if you have enough data, so they are capable of modelling much more complex physical systems.
They can also help you to make predictions for different scenarios. So, a typical use case for us is anything that happens in the validation and verification of cars, batteries, prototypes. You can run the prototype for four hours, and then use the model to predict what would happen if you ran it for another 20, and see whether you liked the results. A lot of people then see this test doesn’t make sense because there’s going to be a failure three hours from now, so I’m just going to reboot it now, and that’s how they’re going to save time.
You start testing completely differently, because you have this machine learning augmentation of ‘let’s try this different scenario that I haven’t looked at’. In vehicle dynamics you can drive on one track, and then predict how the car would behave on a different track without having to actually go there.
How did the partnership with BMW come to be?
BMW came about because they have the BMW start-up garage, which is a start-up scouting organisation. They go through the market, and they try to find new technologies and slot them in.
The start-up garage found us and somebody in vehicle safety said ‘we’re trying to understand when people break their legs and crash tests’. With the simulation models it’s complex; it’s 30,000 components hitting a wall at like 60 miles an hour, so it’s impossible to make sense of the data. BMW used that crash test database plus machine learning to understand how it works with the goal that if you have everyone saved in the crash, you can get a five-star safety rating and then you can remain the premium brand and market leader which is important to BMW.
This is very typical for big company like BMW. They already have thousands of crash tests performed, so they just want to leverage this legacy data. This is not something a Tesla could do because they haven’t crashed thousands of cars yet.
What are some of the other key benefits offered from this technology?
The modelling aspect gives a better understanding of modelling complex equipment, smarter equipment, I think that is the first one.
On top of that, where we see ourselves slotting in more and more – and that’s even changed over the last couple of years – is that we feel like there are many large existing engineering companies and they have a huge amount of existing data already. They have conducted thousands of crash tests, so they have millions of hours of driving and customer feedback, and they just want to make sure that this legacy data can be a competitive advantage – we can help.
These days, we’re moving very, very fast into new directions with EVs, with hydrogen fuel cells and hydrogen car development. Those are all things no-one has ever done before, even with autonomous driving, so people are trying to collect a lot of data on something nobody understands yet. The goal is to make sense of it as quickly as possible, and come up with a clever system that can sort of facilitate or even make decisions.
There’s a nice impact story that Honeywell have released. They really showed how you can go faster, because at the end of the day you really just care about getting a good product to market as fast as you can. What they essentially did is they built a prototype of a gas metre, ran it for 10,000 hours, and then built a machine learning model on top of this data to optimise its performance. This process of prototyping using AI to understand, optimise and calibrate the system, and running it out was three months faster than the original workflow of doing it. Originally you would have a physicist trying to understand what’s going on there, he would build a fairly advanced model, then we try to understand does this model actually work against test data?
It’s more of a black box approach because the machine learning model does it but it’s a lot faster, and it can be powerful when you have a lot of data.
Looking into the future, if we were to have this interview again in a few years’ time, what will have changed?
Right now, the whole AI landscape is changing at an incredible speed. Over the last couple of years, it’s been the innovators, the early adopters who have started solving problems with AI. The AI capabilities that we’ve had were fairly basic – but the capabilities that we’re getting now are actually quite impressive.
I think the number of decisions that you can make with AI is going to be significantly bigger. I think most people will have woken up to the fact that AI is a part of everyday decision making.